Quick Links
The Geo-Mathematical Imaging Group (GMIG) at Rice University has positioned itself as a leading research lab at the intersection of mathematics, geomechanics, and machine learning. The group’s work on earthquake dynamics, planetary exploration and mathematics has supported the discovery of new techniques in carbon sequestration and monitoring, geothermal energy, hydrogen storage, and forecasting. Under the direction of Maarten de Hoop, GMIG has pivoted from an initial interest in exploration seismology for hydrocarbons to focus on key elements of the academic and industrial effort to mitigate climate change.
GMIG spans the full range of (planetary) seismology, combining theoretical research with practical applications of algorithmic development and data processing. A foundation in mathematics, including harmonic analysis, differential geometry, algebraic geometry, and numerical linear algebra, has underpinned recent expansions into deep learning and seismic forecasting. GMIG’s various areas of interest are distinct but complementary, forming an integrated approach that has enabled breakthroughs that would be unobtainable within a narrower framework.
GMIG began publishing research in 2007, focusing primarily on seismic wave equations and imaging. By the mid 2010s, its research had expanded to include a wider range of topics and applications, most notably machine learning and inverse problems. This period also marked a shift in focus from fossil fuels to renewable energy, which continues to inform the direction of GMIG’s current projects.
Presently, GMIG takes an in-depth view of seismicity, flow, geomechanics, and inverse problems, paving the way for innovations in sustainable energy technology. Leveraging its location in Houston, Texas, the heart of America’s energy sector, GMIG receives financial backing from corporate partners as well as significant grant funding from the U.S. Department of Energy and the National Science Foundation. Industry leaders in energy and high-performance computing have joined a long list of academic collaborators in recognizing the importance of GMIG’s blended approach to research.
Some of GMIG’s successes include programs in microlocal analysis and seismic imaging, inverse boundary value problems for the Helmholtz equation, and inverse problems for the elastic wave equation. These legacy projects exemplify GMIG’s capacity for major industrial impact in carbon sequestration and beyond. At the 2018 Mathematics and Physical Sciences Annual Meeting of the Simons Foundation, Maarten de Hoop’s presentation Earth Inside Out detailed how GMIG’s new methods of seismic data acquisition have fueled advances in planetary dynamics, chemistry, and mineral physics. Improved seismic sensors have shed light on previously unseen views of Earth’s interior, unlocking data of equal interest in academia and the private sector.
Rice University’s engagement in energy transition research, including nuclear fusion, wind energy, and hydrogen fuel, has been bolstered by GMIG’s expertise in geophysics. Carbon sequestration and monitoring, and geothermal energy sources, hold significant promise for the future of sustainability. GMIG is deeply involved in research in these areas which has attracted attention from leaders in the energy transition.
With continued success developing technologies in EOR/EGR, carbon sequestration and monitoring, geothermal energy, deep learning, forecasting, uncertainty quantification, decision making, and data science, GMIG has found practical applications for its research in an increasingly wide range of fields. Rapid gains in the areas of machine learning, deep learning, and geoscience have made the lab a sought-after destination for work on earthquakes and planetary missions. Proceeding into the future of sustainability with broadening applications and influence, GMIG is well equipped to form new partnerships for high-impact research.
The Geo-Mathematical Imaging Group (GMIG) at Rice University is a highly respected source of research that synthesizes elements of mathematics, geomechanics, and machine learning. This interdisciplinary approach underpins an extensive body of published work that has found purchase in fields ranging from earthquake dynamics to planetary missions. Within the group’s broad range of interests and applications, key points of emphasis over the last three years include distributed acoustic sensing (DAS), deep learning, and inverse problems. GMIG has leveraged the advantages of its location amid Houston’s thriving energy sector, partnering with industry leaders to forge a path toward a sustainable future. Under the direction of de Hoop, GMIG has pivoted from an initial interest in exploration seismology for hydrocarbons to focus on carbon sequestration and its potential to mitigate climate change.
Math + X Symposium on Matter Under Extreme Conditions in Solar Giants and Exoplanets, Inverse Problems and Deep Learning
Upcoming Lecture series in Spring 2022 on five topics in geo-and space sciences.
Dr. de Hoop’s laboratory is seeking students interested in earning a doctoral degree in either mathematics, machine learning or Earth sciences.
Dr. de Hoop’s laboratory is seeking postdoctoral researchers with a doctoral degree in either mathematics, machine learning or Earth sciences.
Math + X Symposiums
Check to see our upcoming events!
GMIG hosts a series of lectures including the Frontier series, in tandem with both the ECE and Ken Kennedy Institute Lecture Series
Departmental colloquium talks, including an upcoming GMIG series in the Spring Semester of 2022
‘Clustering earthquake signals and background noises in continuous seismic data with unsupervised deep learning', Nature Comm. 11 (2020) 3972, doi:10.1038/s41467-020-17841-x, L. Seydoux, R. Balestriero, M. de Hoop, M. Campillo and R. Baraniuk View |
‘Machine learning for data-driven discovery in solid Earth geoscience', Science 363 (2019) 6433, doi:10.1126/science.aau0323, K. Bergen, P.A. Johnson, M.V. de Hoop, and G.C. Beroza View |
‘Compositional heterogeneity near the base of the mantle transition zone beneath Hawaii', Nature Comm. 9 (2018) 1266, doi:10.1038/s41467-018-03654-6, C. Yu, E.A. Day, M.V. de Hoop, M. Campillo, S. Goes, R.A. Blythe and R.D. van der Hilst View |
Software
![]()
|
![]()
|
![]()
|
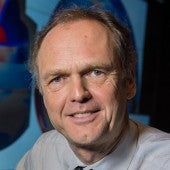
Simons Chair in Computational and Applied Mathematics and Earth Science
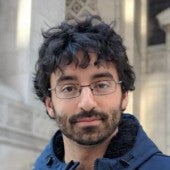
Simons Postdoctoral Fellow, Computational and Applied Mathematics
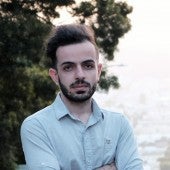
Graduate student, Electrical and Computer Engineering
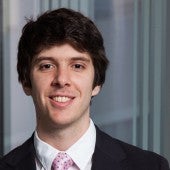
Assistant Professor in Electrical and Computer Engineering
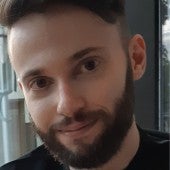
Postdoctoral Research Associate, Computational and Applied Mathematics
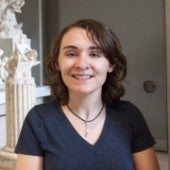
Graduate Student, Earth Science
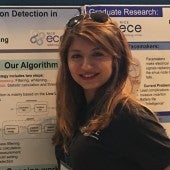
Graduate Student, Electrical and Computer Engineering
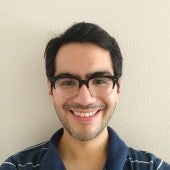
Graduate Student, Computational and Applied Mathematics
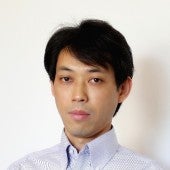
Professor, Mathematics, Computer Science
The GMIG industry project was initiated in 2007 by Professor Maarten de Hoop with the broader purpose of advancing the understanding of our planet’s subsurface and developing fundamentally new technologies in exploration seismology. Over the years, the GMIG has expanded its scope into monitoring, EOR and CO2 sequestration, as well as geothermal energy. Based at Rice University, GMIG’s contributing faculty span the Departments of Mathematics, Electrical and Computer Engineering, Earth Environmental and Planetary Sciences, and Computational and Applied Mathematics. Core GMIG research is primarily sponsored by the partner companies of the Geo-Mathematical Imaging Group, with additional complementary projects funded by the National Science Foundation and other sources. We are deeply indebted to the Simons Foundation for funding under the Math + X program which continues the advancement of our fundamental research into new frontiers. In 2018, with the support of the Simons Foundation, we hosted the Math + X Symposium on Data Science and Inverse Problems in Geophysics, which marked a pivotal move of our group into deep learning and geophysics intertwined collaborations.
For companies looking to create revolutionary products, identifying and accessing cutting edge, exploratory research is the key to competitive advantage. With the shifting importance of human capital over financial capital in the developing Information Age, research universities have proven to be critical partners to innovation success. Return on academic research investment is high, estimated to be between 20% - 40%. Access to qualified talent, projected to be a critical industry bottleneck in data science technologies and analytics, creates an additional incentive: partnering with universities ensures a conduit to highly skilled expertise. According to Harvard Business Review, companies find the following advantages in forming long-term collaborations with universities: Rice University is a private research university in the heart of Houston, Texas, and is consistently ranked among the nation’s top 20 universities by U.S. News & World Report. Founded in 1912, Rice is known for its wide range of research, including fields such as nanotechnology, engineering, architecture, physics, biosciences and social sciences. Rice is home to the James A. Baker III Institute for Public Policy, a globally influential policy think tank.
The GMIG runs the annual Math + X Symposia, which are funded by the Simons Foundation under the MATH + X program to encourage novel collaborations between mathematics and other disciplines in science and engineering. Engineering has been a central part of the Rice University’s focus since its founding. The George R. Brown School of Engineering has earned a place among the top engineering schools in the country by partnering with sciences, humanities, social sciences, architecture and business to develop engineering solutions in an increasingly complex world. Departments, Institutes and Centers within the School of Engineering contribute to Houston’s role as a center for the energy industry, medical research, space exploration and the city’s growing high-technology sector. The Ken Kennedy Institute for Information Technology is dedicated to the advancement of research in the fields of computing, data science and information technology and annually hosts the Oil and Gas High Performance Computing Conference and Rice Data Science Conference. Seeding of early-stage research. Instead of just monitoring early stage research at universities and investing when products of interest emerges, smart companies increasingly seed in areas of interest to them. Continuous and intimate connection to early stage research accelerates the translation of that research into new products that drive economic growth.